From transforming healthcare to revolutionizing finance, Artificial Intelligence (AI) and Machine Learning (ML) workloads have become crucial drivers of innovation. These data-centric tasks, however, demand immense computational power, making GPU deployment a game-changer. VMware’s Tanzu Kubernetes Grid Service (TKGS) equipped with vGPU support emerges as the ideal solution, enabling AI/ML workloads to harness the full potential of virtualized GPUs.
In this blog post, we will see how to deploy AI/ML workloads on TKGS clusters with vGPU support.
Prerequisites
Before we dive into the deployment process, ensure you have the following prerequisites in place:
- Up and Running TKGs: Tanzu Kubernetes Grid Service must be operational on your vSphere environment. (Check from vCenter -> Workload Management.)
- GPU Installed on Host: Your ESXi hosts should have compatible NVIDIA GPUs installed and recognized by the system.
- NVIDIA VIB Installed on ESXi: Verify that the NVIDIA driver is installed on ESXi (esxcli software vib list | grep NVIDIA)
For detailed instructions on installing the NVIDIA VIB on ESXi hosts, you can refer to the official VMware documentation provided here.
My Environment
vCenter: 8.0.1
ESXi: 8.0.1
GPU: NVIDIA A10
Deployment
Step 1: Create a Custom VM Class with a vGPU Profile
Login to vCenter -> Workload Management -> Services -> Click Manage under “VM Service”
Click “Create VM Class”
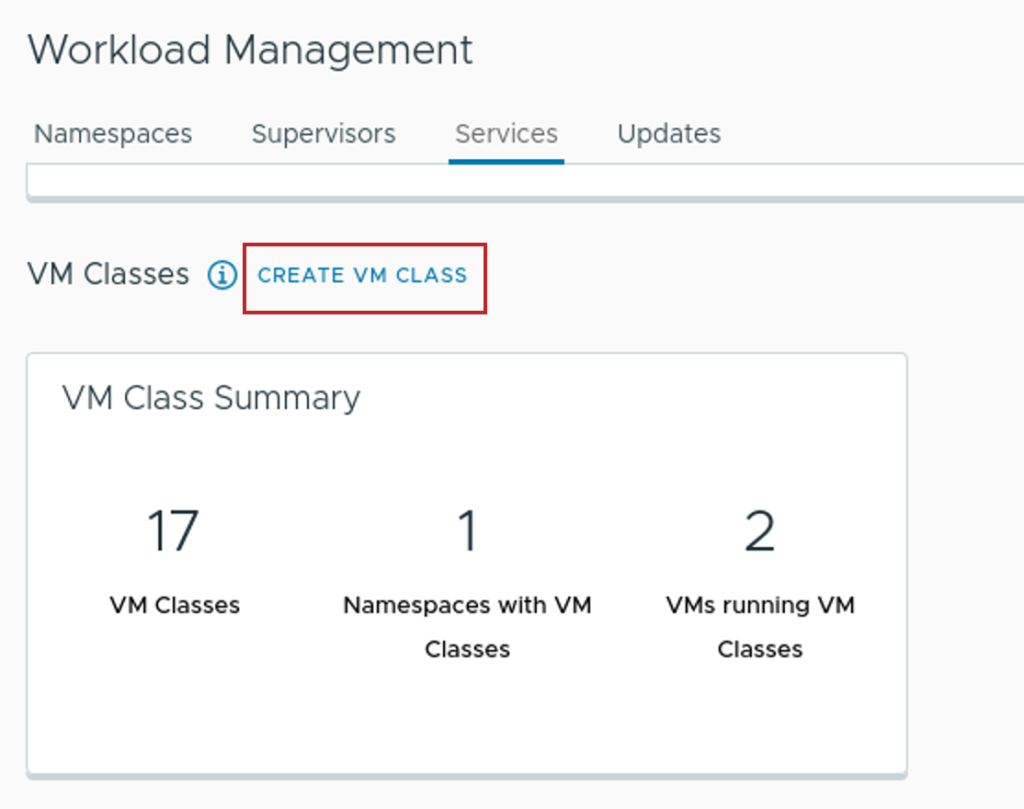
Please note we have to select PCI Devices (100% Mem reservation)
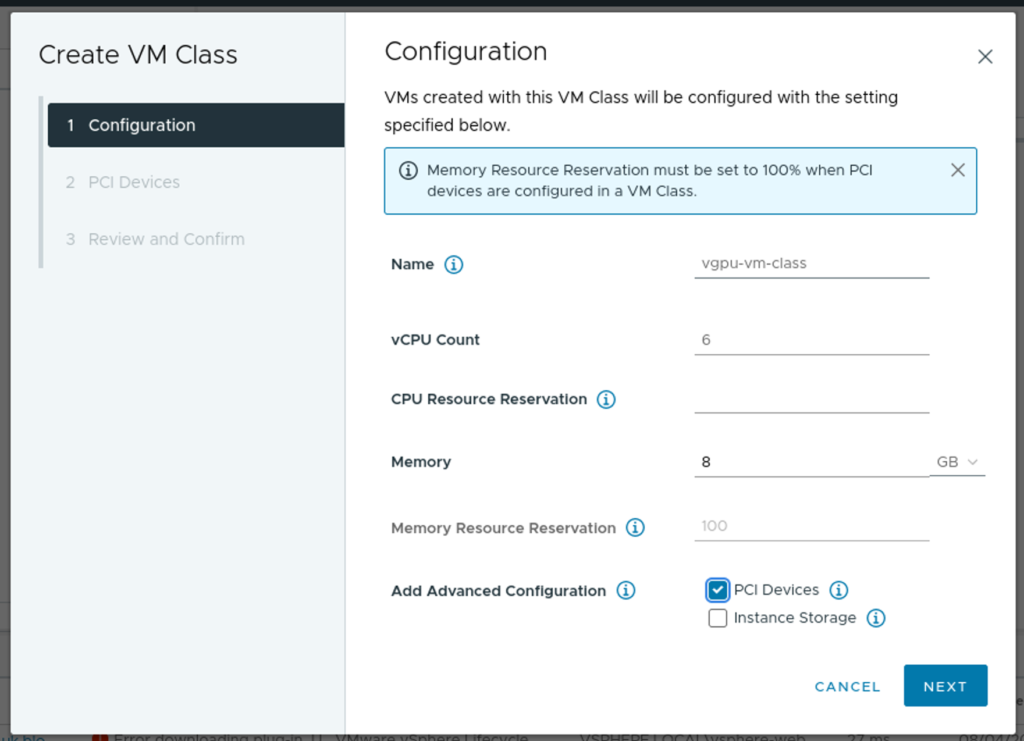
Select your PCI Device
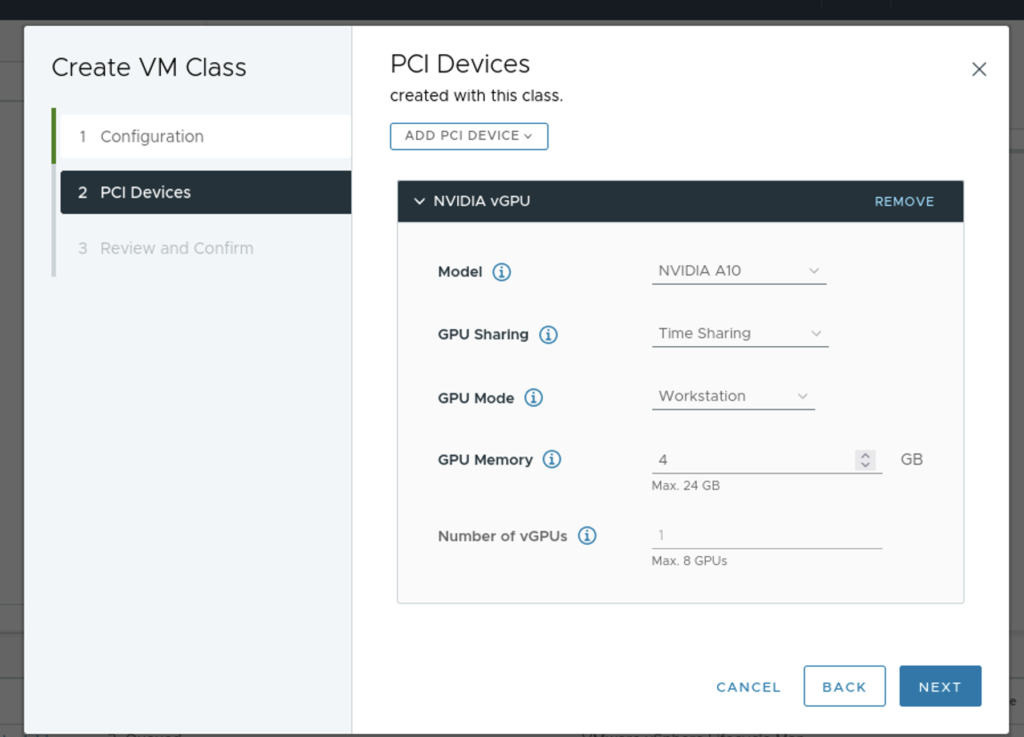
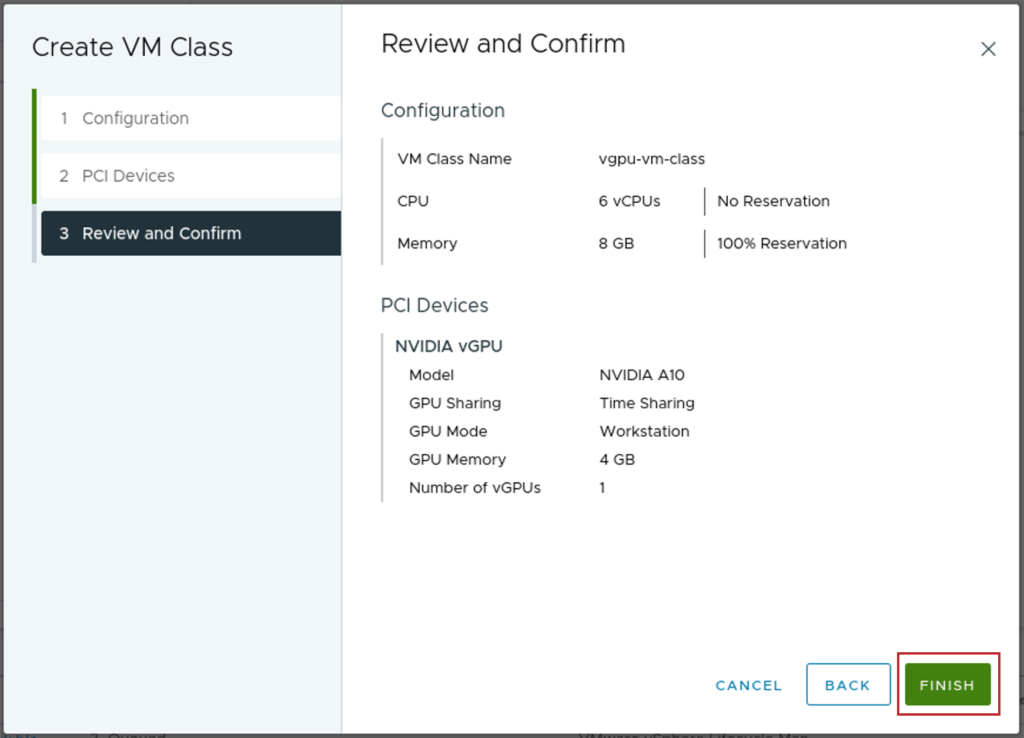
Verify that the new custom VM Class is available in the list of VM Classes.
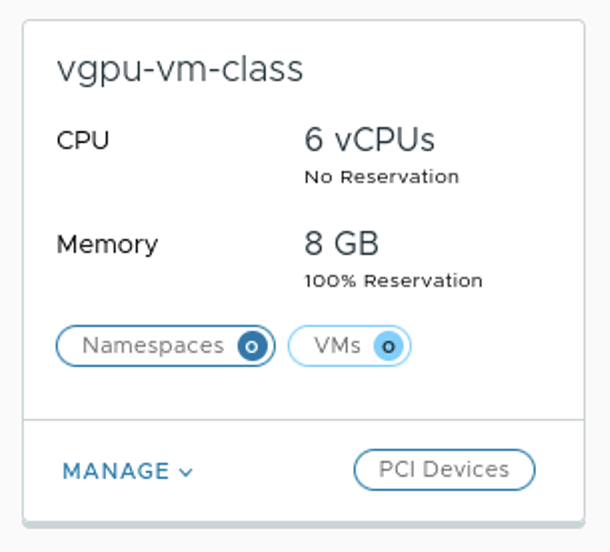
Step 2: Create a Namespace and add VMclass and Content Library
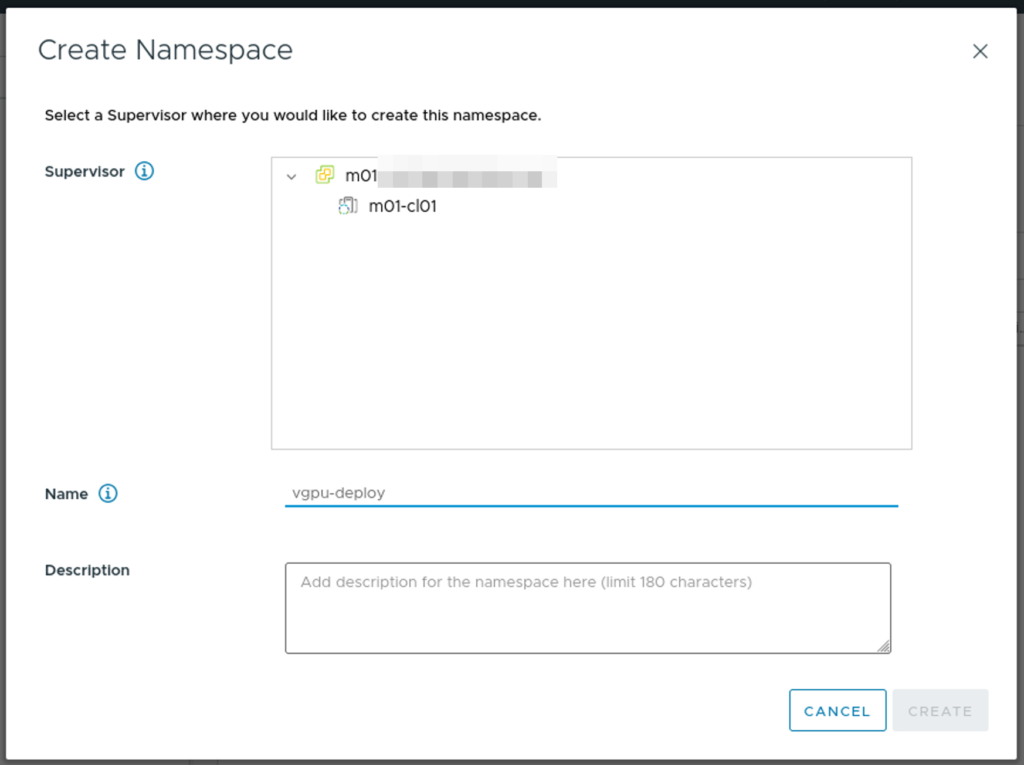
Add the custom VM Class which we created in Step 1 and your TKGs Content Library
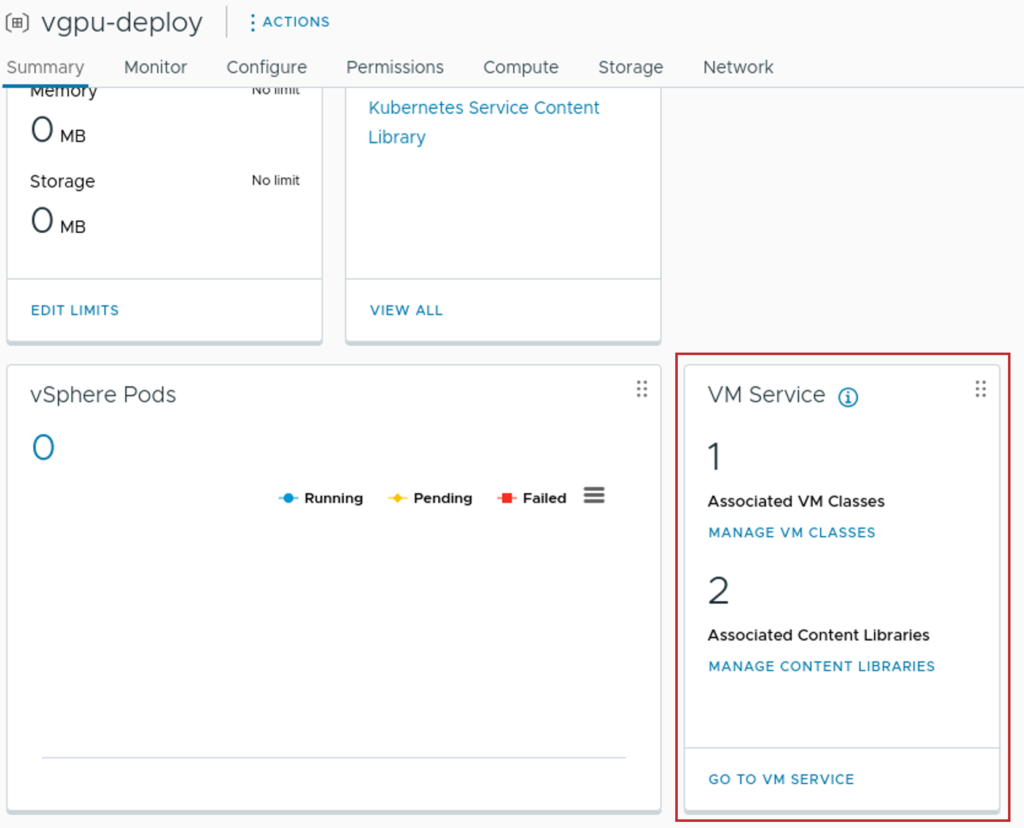
Step 3: Install kubectl vSphere and login to Supervisor Cluster
curl -LOk https://Control Plane Node Address/wcp/plugin/linux-amd64/vsphere-plugin.zip
unzip vsphere-plugin.zip
mv -v bin/* /usr/local/bin/

kubectl vsphere login --server=IP-ADDRESS --vsphere-username USERNAME --insecure-skip-tls-verify

Step 4: Prepare yaml to deploy the vGPU cluster
Switch to the context we created in Step 2


kubectl config get-contexts
kubectl config use-context vGPU-NAMESPACE
kubectl get virtualmachineclassbindings
Create a yaml file to deploy the cluster
apiVersion: run.tanzu.vmware.com/v1alpha2
kind: TanzuKubernetesCluster
metadata:
#cluster name
name: tkgs-cluster-gpu-a10
#target vsphere namespace
namespace: vgpu-deploy
spec:
topology:
controlPlane:
replicas: 1
#storage class for control plane nodes
#use `kubectl describe storageclasses`
#to get available pvcs
storageClass: tkgs-sp
vmClass: guaranteed-medium
#TKR NAME for Ubuntu ova supporting GPU
tkr:
reference:
name: 1.22.9---vmware.1-tkg.1
nodePools:
- name: nodepool-a10-primary
replicas: 1
storageClass: tkgs-sp
#custom VM class for vGPU
vmClass: vgpu-vm-class
#TKR NAME for Ubuntu ova supporting GPU
tkr:
reference:
name: 1.22.9---vmware.1-tkg.1
- name: nodepool-a10-secondary
replicas: 1
vmClass: vgpu-vm-class
storageClass: tkgs-sp
#TKR NAME for Ubuntu ova supporting GPU
tkr:
reference:
name: 1.22.9---vmware.1-tkg.1
settings:
storage:
defaultClass: tkgs-sp
network:
cni:
name: antrea
services:
cidrBlocks: ["198.51.100.0/12"]
pods:
cidrBlocks: ["192.0.2.0/16"]
serviceDomain: managedcluster.local
Step 5: Deploy the cluster
kubectl apply -f vgpu-cluster.yaml

TKC cluster with vGPU has been deployed. Hope this helps!